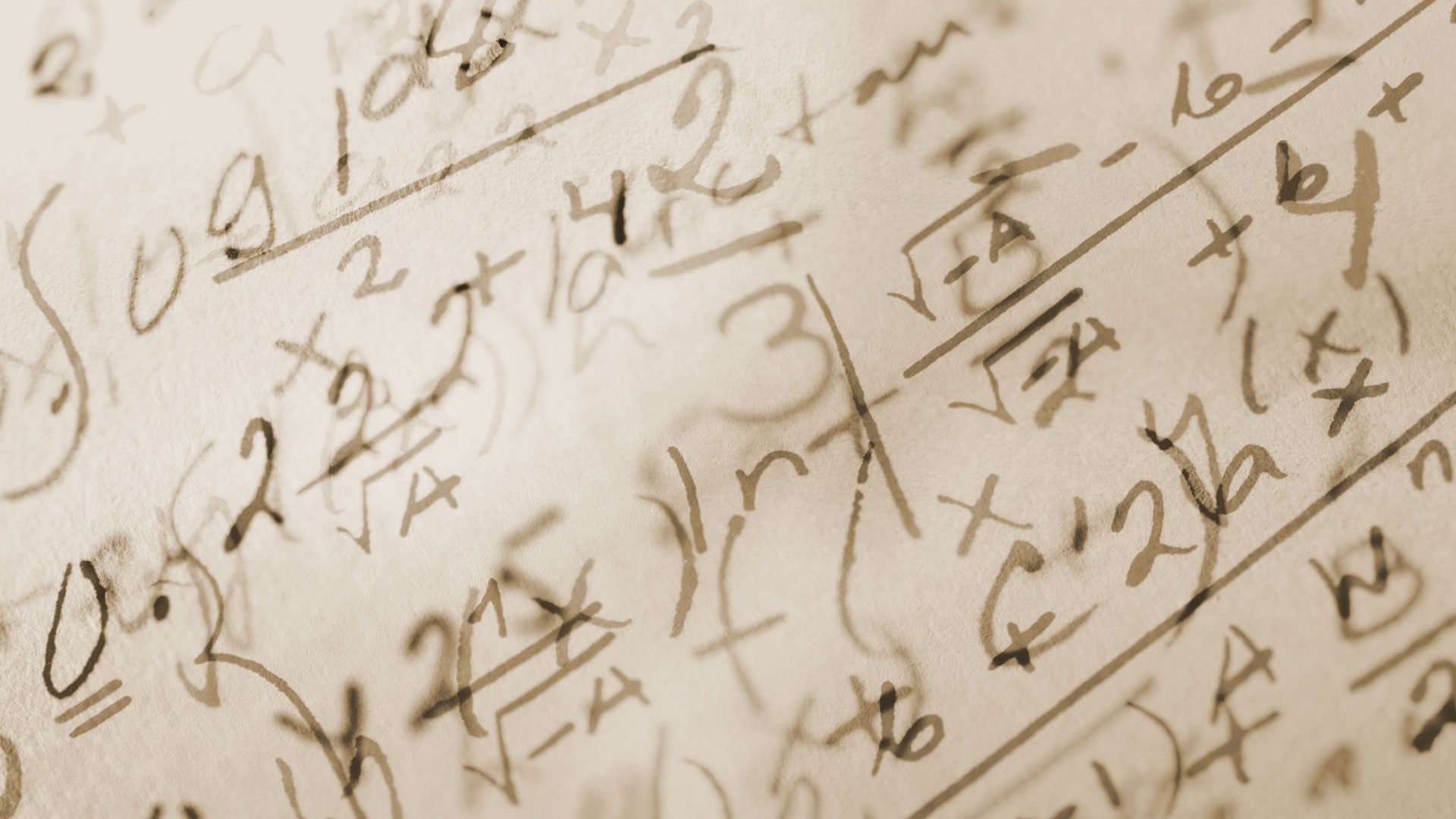
When ChatGPT hallucinates and starts concocting answers that have no basis in reality, this is an example of an artificial intelligence system behaving in a way that cannot be explained. ChatGPT is a large language model (LLM) based on a deep learning algorithm. Deep learning is a variety of machine learning – and both are forms of artificial intelligence.
In order for artificial intelligence to be based on a deep learning algorithm, it must first be trained by humans. This is typically done by ‘feeding’ the system with large volumes of data while also feeding it with the answer sheet in the form of the solutions we want the artificial intelligence to find in future. In the world of research, many researchers use deep learning systems to identify connections and patterns in large data sets – for example when tracing links between genes and diseases.
Loss of control
Following the training phase, the artificial intelligence is unleashed to find the right solutions based on new data while also simultaneously learning from the data that it encounters. This is why this type of artificial intelligence is called self-learning. However, while the algorithms that allow the artificial intelligence to successfully complete its task may have been developed by us humans, we do not necessarily understand how it completes the task, according to Søren Hauberg, Professor at DTU Compute. He elaborates:
“You could say that we have given the artificial intelligence the freedom to select any method of its choosing in order to complete its tasks. This means that we can’t always account for what is taking place and how it reaches the solutions that it then presents us with – these processes are often hidden from us.”
The hidden processes contained in self-learning artificial intelligence systems are known as black boxes.
“A black box represents a loss of control and there are situations where that loss of control is not acceptable. If the artificial intelligence in question is performing a task such as controlling a robot on a car assembly line, then it simply isn’t acceptable for us not to have a full grasp of how it moves from A to B – dangerous situations may arise if it behaves unpredictably. This is one of the reasons why we stand to gain a lot from finding out what is going on inside black boxes,” Søren Hauberg says.
PROFESSOR SØREN HAUBERG DTU COMPUTEA black box represents a loss of control and there are situations where that loss of control is not acceptable.
Pillows in saucepans
Søren Hauberg has found mathematical methods that provide us with a glimpse inside the black box. His approach is to examine the potential errors that can occur when a large data set is compressed. The artificial intelligence system has to compress data in order to filter out irrelevant information.
“Data comprises not only the information we need, but also errors in measurement and other irrelevant information we refer to as noise – and all of this noise is removed through compression. In other words, a type of data filtering takes place where the wheat is separated from the chaff,” says Søren Hauberg.
However, unexpected correlations in the data can occur during the compression process, which leads to the artificial intelligence finding incorrect patterns and then outputting the wrong result.
Søren Hauberg explains the whole mess with a home-moving analogy:
“Imagine you’re moving home and have to pack your whole house into boxes. In order to make the best possible use of your boxes, you put your pillow inside a saucepan. If someone who doesn’t know anything about how we live were to draw conclusions from that box, they might well believe that we keep our pillows in the kitchen or saucepans in the bedroom. Yet the two things have nothing to do with each other – and there is no correlation between them. Packing them into the box like that was simply the smart thing to do. The same is true for data compression. There are lots of ways that artificial intelligence can compress data, and if the technology then tries to establish what the underlying patterns are in the data that has been ‘packed away’, there’s a risk it draws the wrong conclusions.”

Professor Søren Hauberg has received an additional EUR 2 million in early 2024 to continue his research to explain the black box phenomenon in artificial intelligence. Photo: Hanne Kokkegård
200-year-old mathematics
In his research, Søren Hauberg has therefore sought out mathematical formulas that correct for the errors that can occur in data sets during compression.
“As part of our basic research, we’ve found a systematic solution that allows us to theoretically walk backwards so we can keep track of which patterns are grounded in reality and which ones have been fabricated by the compression process. When we are able to separate these, we humans can gain a better understanding of how artificial intelligence works – while also being reassured that the artificial intelligence isn’t listening to false patterns.”
The mathematical formulas utilized by Søren Hauberg and his colleagues are hardly brand new – they were in fact developed in the 19th century for use in cartography.
“When they tried to draw maps, they were seeking to transfer information from a three-dimensional sphere to a two-dimensional surface. This created a number of distortions: for example, the land masses are not in accurate proportion to one another meaning that Greenland appears to be much bigger than Africa. The mathematical formulas that correct for these distortions can also be used in our research examining the black boxes of artificial intelligence,” says Søren Hauberg.
May prevent ChatGPT’s hallucinations
The researchers have now made sufficient progress that they are able to look inside the black boxes of artificial intelligence models that use data compression.
“These models are typically used in research when researchers try to find out whether there are any underlying patterns in the data they are working with. The prevention of incorrect conclusion is directly relevant to the working processes of academia,” says Søren Hauberg.
He adds that their work is still unable to correct errors that are found in artificial intelligence systems such as ChatGPT. However, he notes that the researchers’ work has the potential to do so in future.
“We’d love to be able to explain why a chatbot like ChatGPT hallucinates. We can’t do that yet, but perhaps we will be able to in a couple of years’ time,” says the professor, who received a in early 2024 to support his continued research into black boxes.