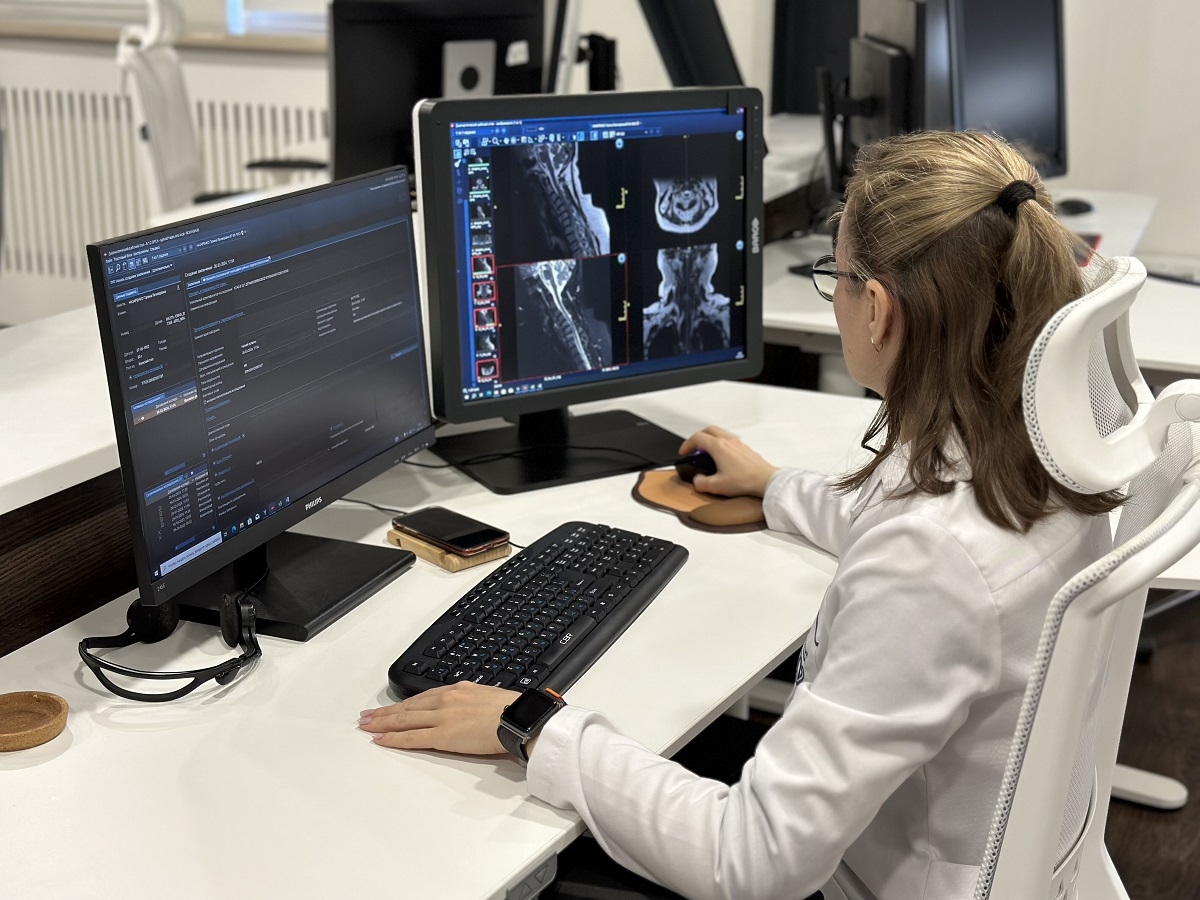
This advanced technology enables the detection of disc protrusion, herniation, and spinal stenosis, hitherto confined to the lumbosacral region, utilizing artificial intelligence algorithms. According to Anastasia Rakova, Deputy Mayor of Moscow for Social Development, this neural network expedites the process of conducting crucial measurements, significantly accelerating diagnosis.
Rakova further emphasizes the city’s ongoing commitment to integrating AI into medicine, highlighting the broadened scope of AI utilization within Moscow’s healthcare system. The Deputy Mayor underscores the important role of AI in expediting diagnosis by swiftly assessing stenosis percentages, measuring protrusion and herniation sizes, and evaluating spinal canal dimensions. With over 50 AI services currently operational in Moscow, capable of identifying 37 different diseases, the city stands at the forefront of AI-driven medical innovation.
Moreover, Moscow extends its technological expertise to other regions of Russia, offering access to a platform that enables nationwide healthcare professionals to leverage the capital’s cutting-edge algorithms for processing radiological study results.
Yuri Vasilev, Senior Consultant for Radiology and CEO of the Centre for Diagnostics and Telemedicine of the Moscow Department of Health, underscores the transformative impact of AI in radiology, particularly in MRI examinations. Yuri Vasiliev clarifies how the integration of digital services streamlines diagnostic processes, empowering specialists to efficiently diagnose conditions such as protrusion, herniation, and spinal canal stenosis. He further introduces the maturity matrix—a pioneering approach developed by the Centre Diagnostics and Telemedicine – to assess and compare AI-driven diagnostic solutions, ensuring the deployment of rigorously validated AI services tailored to radiologists’ needs.
The inception of smart algorithms in 2020 marked a significant milestone in Moscow’s healthcare landscape, ushering in an era of computer vision technology adoption. Integrated into the unified radiological information service EMIAS, these neural networks annotate medical images with color-coded indicators, identifying potential pathologies and providing clinically relevant measurements essential for reporting.